Developing Embedded AI Systems
Embedded artificial intelligence (AI) will enable new, inexpensive, and low power AI solutions that are not possible using cloud-based AI technologies alone. The edge AI chip market is growing much faster than the overall chip market, with the number of edge AI chips to be sold in 2024 estimated to be 1.5 billion. Embedded AI requires knowledge and skills beyond traditional embedded systems, data science, and machine learning (ML). It requires knowledge of devices, sensors, and advanced, near real-time signal processing methods for video, audio, motion, or other signals. Specialized software tools and frameworks are required to develop embedded AI applications.
This program provides the knowledge and skills required to take advantage of this next major shift in technologies and the related growth in job demand. The program explores the specialized tools, frameworks, technologies, platforms, and methods used to create exciting new embedded AI devices. Study TinyML – the field of applying ML technologies to embed AI in resource-constrained devices. Discover how complex embedded AI applications work on smartphones, drones, and other devices that have constrained processing, memory, power, and other resources. Investigate the signal processing methods and ML models behind important applications that process video, audio, motion, and other signals. Explore how ML frameworks are used to create these applications and use these with embedded AI hardware in hands-on projects. Learn how to choose the right hardware, development tools, and software components for an application. Examine the tradeoffs needed to make decisions about the mix of AI processing that needs to be done on the device and in the cloud. Apply what you have learned and use cutting-edge devices, sensors, signal processing, TinyML methods, and embedded ML frameworks to create an embedded AI device.
Who Should Enroll
This program is ideal for those who wish to learn about the field of embedded AI, acquire the specialized skills needed to create embedded AI solutions, explore new uses, solve problems using constrained edge devices, gain competitive business advantage, and expand career options. The curriculum is designed for embedded systems professionals, software engineers, electrical engineers, computer engineers, computer scientists, data scientists, data engineers, and machine learning scientists.
Program Benefits
- Use hands-on examples and activities to explore embedded AI applications, technologies, and highly specialized embedded ML software frameworks
- Explore the details of embedded ML models (including deep learning neural networks) behind some important applications such as object recognition, wake word processing, and gesture detection
- Review the steps required to develop and deploy embedded ML models
- Understand the unique challenges posed by the deployment of ML models on resource constrained devices in TinyML applications
- Use cutting-edge sensors, devices, signal processing, TinyML methods, and embedded ML frameworks to create an embedded AI device
- Collect real-world sensor data, train, and validate ML models, optimize the model for deployment on a resource-constrained device, and deploy the model to your hardware
- Explore features of devices that may be used to run embedded AI, including new low power microcontrollers which incorporate neural network accelerators that will enable new battery powered applications to execute complex ML models
- Explore current trends and what may be on the horizon for embedded AI
Earn an Alternative Digital Credential
Successful completion of a qualifying assignment within select course(s) offers you the opportunity to earn an Alternative Digital Credential (ADC). Also referred to as a digital badge, an ADC is a virtual record of specific skills and competencies you have acquired and provides a verifiable way for you to share your educational achievements with employers, colleagues and others via social platforms like LinkedIn or Facebook.
Explore the criteria necessary to earn an ADC in:
- Embedded Artificial Intelligence: Basics
Applicable course: Introduction to Embedded AI (completed after 12/22/21*)
Learn more about Alternative Digital Credentials and how they can help you demonstrate your commitment to professional development and distinguish yourself in a competitive job market.
*Courses completed prior to indicated dates are not eligible for a badge.
Eligibility and Requirements
Apply to become a candidate for the program: A Declaration of Candidacy is required to establish candidacy in this program. Complete the application and pay the application and candidacy fee of $125 (non-refundable). Learn more about the benefits of becoming a candidate. Candidacy is not required to enroll into individual course in a program.
A candidate in the program is awarded a specialized studies certificate upon the successful completion of three (3) required courses totaling 9 units, each with a letter grade of “C” or better. All requirements must be completed within five (5) years after the student enrolls in their first course. To receive the Certified Digital Certificate after completing all the program requirements, students must submit the Request for Certificate to initiate the certificate audit process. Students not pursuing the certificate are welcome to take as many individual courses as they wish.
Specialized Studies
Enroll and pay for courses as you go.
Have Questions?
Talk to an enrollment coach
Call: (949) 824-5414
Monday - Friday, 9am - 4pm (Pacific)
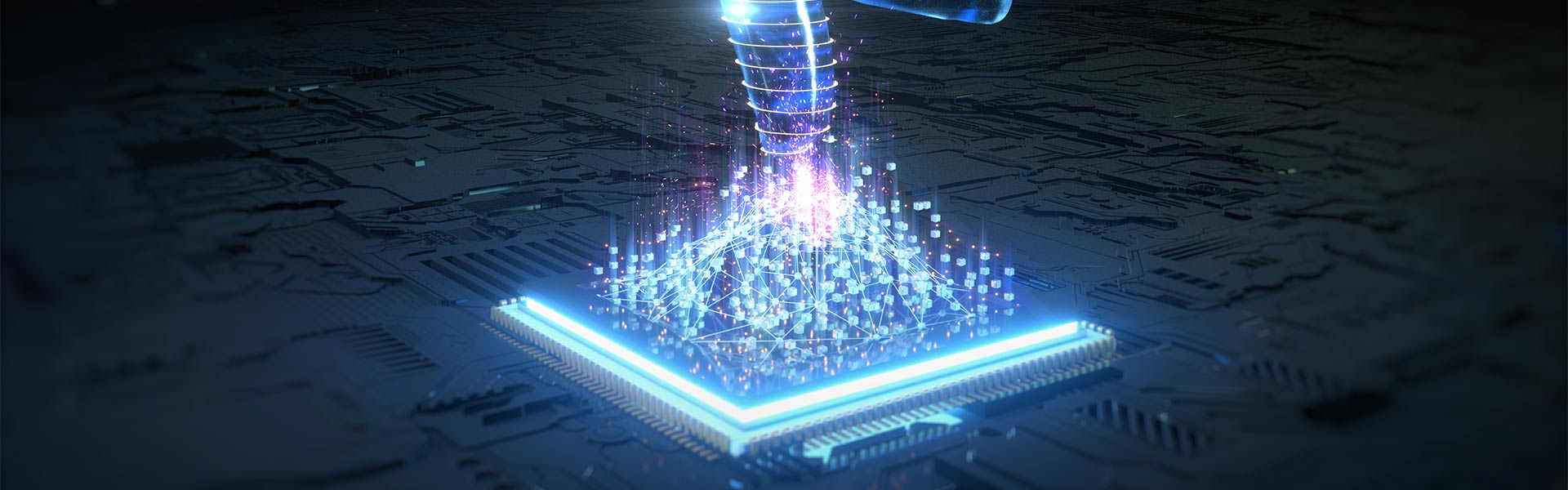
Course Schedule
Course schedules are subject to change. Individual courses may be taken without enrolling in the full program.
Career Advancement Network
DCE is proud to offer our learners, candidates and graduates valuable career resources, including personalized career coaching, mentorship, online technology tools, live webinars, and more.